Mastering the Correlation Coefficient: A Guide
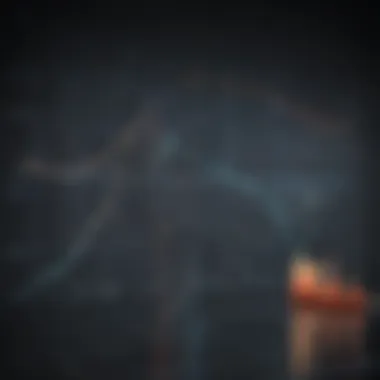
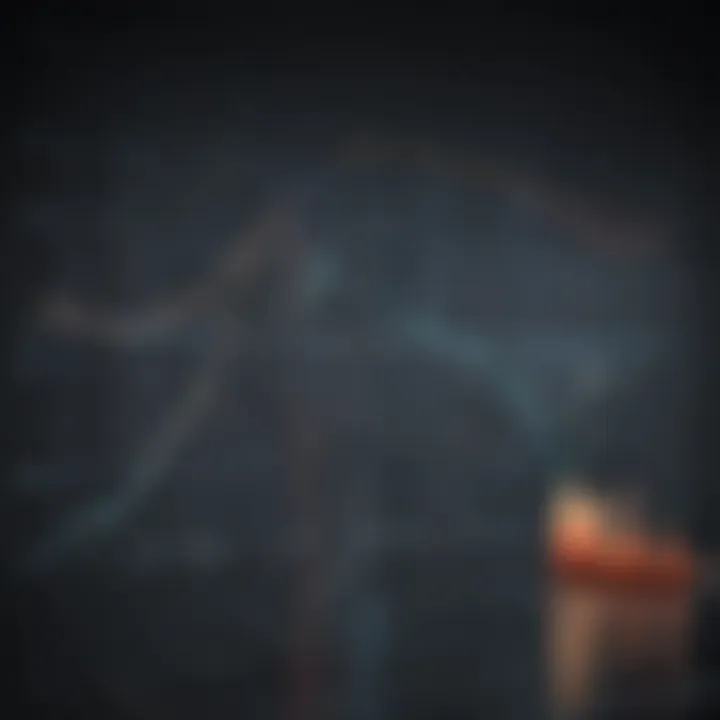
Intro
Understanding the correlation coefficient is pivotal in the realm of finance and data analysis. It serves as a statistical gauge for evaluating how two variables move in relation to each other. This coefficient, often expressed as a value between -1 and 1, can unveil crucial insights, especially for those eager to make informed investment decisions. Knowing how to calculate and interpret this coefficient can make a significant difference in an investor's strategy, aiding in the identification of market trends, risk mitigation, and portfolio optimization.
The power of correlation analysis lies not just in the numbers but in what they signify about the relationships between different financial variables. Whether one is examining the correlation between a stockâs performance and market indices or assessing the interplay between economic indicators, understanding these relationships can unveil hidden patterns that are otherwise difficult to discern.
In the sections that follow, we will meticulously explore the various dimensions of the correlation coefficient, highlighting its relevance and utility in financial decision-making. Weâll also delve into distinct methodologies for its calculation, pitfalls to be mindful of, and real-world applications that underscore its significance.
As we embark on this journey to master the correlation coefficient, letâs remember: âIn the world of finances, the more you know, the better equipped you are to navigate.â This exploration equips investors and analysts alike with the tools they need to leverage data effectively.
Prolusion to Correlation Coefficient
The correlation coefficient is a foundational piece in the puzzle of statistical analysis. It acts as a key indicator for understanding how two variables are related, offering insights that can influence decision-making across various domains, particularly in finance. Whether one is assessing the relationship between stock prices and market indices, or comparing commodity prices to inflation rates, having a grip on this concept can spell the difference between making astute investments and pulling oneâs hair out in frustration.
By understanding the correlation coefficient, individuals can evaluate whether an increase in one variable suggests an increase or decrease in another. This understanding not only serves investors seeking profitable opportunities but also equips analysts with the necessary tools to create more comprehensive financial models. The relevance of this coefficient lies not only in its application but in its ability to inform strategy and risk assessment.
"Correlation does not imply causation" â an important lesson for any analyst.
Definition and Purpose
The correlation coefficient is a numerical measure that encapsulates the degree of linear relationship between two variables. It ranges from -1 to +1, with these values indicating the strength and direction of the relationship. A score of +1 indicates a perfect positive correlation, meaning that as one variable increases, the other does too. Conversely, a score of -1 indicates a perfect negative correlation, where one variable decreases as the other increases. A score of 0 implies no correlation at all, indicating that the two variables move independently of one another. Understanding this measure is crucial for making informed predictions in finance.
In practical terms, the correlation coefficient serves several purposes:
- Predictive Analysis: Helps in forecasting future trends based on historical data.
- Risk Management: Aids in identifying potential risks, particularly in portfolio management, by indicating the relationships between different assets.
- Benchmarking: Provides a tool for comparing the performance of various securities against market indices or other benchmarks.
Understanding how to calculate and interpret this coefficient is invaluable for both seasoned analysts and budding investors alike.
Historical Background
The concept of correlation has its roots in the early 19th century but gained prominence with the work of Karl Pearson. His research in the late 1800s laid the groundwork for statistical relationships and introduced the most widely used measure of correlationâthe Pearson correlation coefficient.
Pearsonâs work was revolutionary for his time. However, the journey didnât stop there. In the 20th century, Spearman and Kendall further refined correlation metrics. Spearman's Rank Correlation, introduced in 1904, stepped away from the assumption of linear relationships and offered a way to compute correlations based on ranked variables.
As statistical methodologies evolved and became more sophisticated, so too did the application of correlation. In financial contexts, correlation has become a bedrock principle in portfolio management and risk assessment, allowing investors to construct diversified portfolios that balance risk and return.
With the dawn of advanced computational methods and data analytics in recent years, the understanding and calculation of correlation have reached new heights. Today, accessing massive datasets in real-time allows for a more nuanced approach to correlation analysis, proving to be essential in todayâs fast-paced financial environments.
Types of Correlation
Understanding the various types of correlation is not just a venture into statistical territory; itâs a gateway to grasping the intricate relationships that exist between data points. A well-rounded understanding of correlation types enriches our insights into data, especially in fields like finance, where these relationships can dictate market trends and investment strategies.
Positive Correlation
Positive correlation refers to the scenario where two variables move in tandem, meaning as one increases, the other also rises. This relationship is vital in financial contexts. For instance, consider the relationship between a company's earnings and its stock price. Generally, when a company shows growth in earnings, its stock price is likely to follow suit, reflecting the investor confidence that arises from solid performance.
To put it plainly, a positive correlation can often be interpreted as a harmony between elementsâakin to two dancers moving gracefully to the same rhythm.
- Identifying Positive Correlations: Analysts often look for patterns in historical stock prices or economic indicators. The correlation coefficient will yield a value between 0 and 1, where 1 denotes a perfect positive correlation.
- Practical Applications: Investors often use positive correlations to create portfolios where linked assets can enhance returns. If two stocks show a strong positive correlation, an investor might decide to hold both, believing they will react similarly to market changes.
Negative Correlation
On the flip side, negative correlation implies a seesaw effectâwhen one variable rises, the other falls. Take a classic example from finance: the relationship between interest rates and bond prices. As interest rates rise, existing bond prices typically drop, leading to a negative correlation.
This kind of relationship is crucial for risk management and investment strategies:
- Understanding Risk: Investors recognize that diversifying their portfolios with assets that exhibit negative correlation can mitigate potential losses. In effect, when the value of one asset dips, the other may bolster overall returns.
- Statistical Insight: A correlation coefficient nearing -1 indicates a perfect negative correlation. This quantifiable insight aids in financial modeling and predictive analytics.
Zero Correlation
Zero correlation is intriguing. Here, the movement of the two variables bears no relationship to one another. Imagine the correlation between the sales of ice cream and the number of umbrellas sold on a summer's day. They could rise and fall independently, offering no discernible link logistically.
In the world of finance, not all data sets align perfectly. Recognizing zero correlation can also be informative:
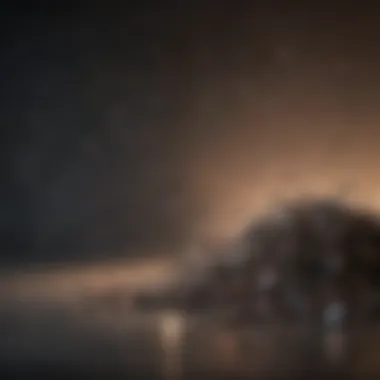
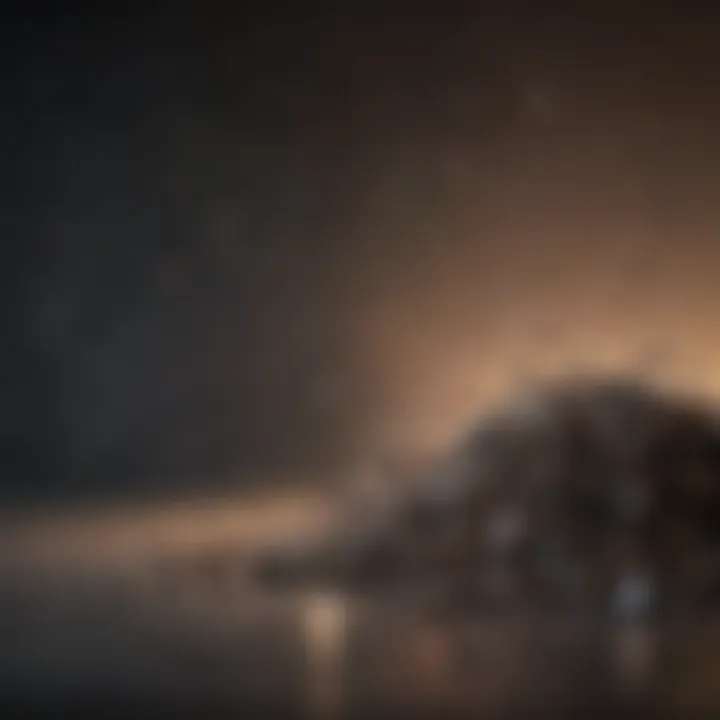
- Investment Decisions: When two assets show zero correlation, they may not affect each other's performance. This independence can foster a more balanced investment portfolio, particularly in volatile markets.
- Misinterpretations: It's crucial, however, to note that a zero correlation does not imply a lack of relationship. Non-linear relationships sometimes exist, but they do not conform to linear correlation metrics.
"Correlation does not imply causation, but it certainly provides clues for deeper examination."
In summary, understanding these types of correlationâpositive, negative, and zeroâenables investors and analysts to draw distinctions in data interpretation and relationship dynamics, essential for strategic decision-making. Their relevance in finance cannot be overstated; recognizing how different variables interact is fundamental in crafting a successful investment strategy.
Mathematics of Correlation Calculation
The mathematics behind correlation calculation is more than just graphs and numbers; itâs the backbone of statistical analysis, helping to quantify relationships between variables. Understanding these mathematical concepts is crucial for anyone looking to dissect complex data sets, especially in finance, where decisions may hinge on the strength and direction of relationships.
Correlation coefficients provide a numerical way to describe how closely tied two variables are. Whether youâre a seasoned analyst or a curious investor, grasping these mathematical foundations helps to better predict outcomes based on historical data. Not to mention, such knowledge empowers one to differentiate between correlational statistics and more simplistic interpretations that may not capture the nuances of real-world data.
Pearson Correlation Coefficient
The Pearson correlation coefficient is the most commonly used method to measure linear relationships between two variables. Its value ranges from -1 to +1. A value of +1 indicates a perfect positive correlation, while -1 indicates a perfect negative correlation. When you find a result near zero, it suggests there is no linear relationship at all.
Let's pull at this concept with a practical example. Imagine measuring the relationship between a companyâs advertising spend and its revenue. The higher the advertising spend, the greater the anticipated revenue, theoretically leading to a strong positive correlation. However, this method assumes the data is normally distributedâif thatâs not the case, the derived coefficient may mislead you.
It's vital to ensure your data meets the necessary conditions, as otherwise, conclusions drawn from the Pearson coefficient can lead to erroneous interpretations.
Spearman's Rank Correlation
Contrasting with Pearson's, Spearman's rank correlation does not necessitate a linear relationship nor requires normal distribution of data. This method assesses how well the relationship between two variables can be described by a monotonic function. In simpler terms, it's about ranking the data rather than using raw numbers.
For instance, if two sets of rankings are examinedâsay one for product quality and the other for sales numbersâyou could employ Spearman's rank to evaluate their relationship. If higher quality consistently ranks ahead of lower quality, you might find a significant positive correlation. Variations in performance are acknowledged better here, offering a wider lens into correlation without getting hung up on the intricacies of data distribution.
Kendall's Tau
Another robust method to consider is Kendall's Tau, which gauges the ordinal association between two quantities. Like Spearman's, it works well with less-than-ideal data distributions. Essentially, Kendall's Tau measures the probability that the ranks of the two variables agree compared to those that disagree.
To illustrate, consider a survey asking customers to rank their satisfaction with two products. The correlation can help determine whether customers who are happy with Product A express similar feelings towards Product B. The chance of agreement in rankings generally lends insight into how similarly the two products are perceived.
Kendall's Tau is particularly beneficial in cases where data includes many ties, as it effectively accommodates duplicates without skewing results. It's a handy tool, especially in qualitative research, to surface relationships that are often ignored.
Understanding the mathematical foundations of correlation is essential in achieving sound results and interpretations.
In summary, the mathematics of correlation is not just an academic exercise; it is a vital skill for financial enthusiasts and individual investors alike. By familiarizing oneself with these methods, you enhance your ability to analyze relationships and make informed decisions based on comprehensive data assessments.
Step-by-Step Calculation Process
Understanding the mechanism of calculating the correlation coefficient is paramount for any financial analyst or investor. This section breaks down the essential stages of the calculation process, providing clarity to what can sometimes be a daunting task. By approaching the calculation in a stepwise manner, one can minimize potential errors and enhance confidence in deriving insights from data. Each step corresponds to a crucial element in ensuring the integrity of the analysis and ultimately, contributing to more informed decision-making.
Data Collection
Data collection serves as the foundation for any analysis. At this stage, itâs vital to gather relevant data that reflects the variables you intend to analyze. This includes, but is not limited to, historical stock prices, economic indicators, or even consumer sentiment measures, depending on what youâre scrutinizing.
When pulling data from diverse sources, such as market feeds, databases, or even manually compiled datasets, a few key practices can streamline this process:
- Consistency: Ensure that the data points are gathered under the same parameters (like time frame, frequency, etc.). For example, if you're analyzing daily returns, stick to daily data across all variables.
- Validity: Use reliable sources. Websites such as en.wikipedia.org or britannica.com can bolster the credibility of the information.
- Comprehensiveness: Aim to collect enough data to build a robust analysis. A wider dataset can refine the results.
Data Preparation
Once data is collected, the next step pivots towards preparation. If you think of it as preheating an oven before baking, data preparation sets the stage for accurate results. Here, the main tasks include:
- Cleaning the Data: Remove any outliers or incorrect entries that could skew results. For example, if one stock price seems wildly off compared to historical prices, itâs likely an error worth correcting.
- Normalizing the Data: Standardize your data sets to ensure they are comparable. This might entail converting stock prices to returns by calculating the percentage change between periods.
This step not only simplifies subsequent calculations but also enhances the reliability of your analysis.
Performing the Calculation
Now comes the moment of truth: performing the calculation itself. Depending on your choice of correlation method, the process can vary slightly. However, the general sequence includes:
- Select the Method: Choose between Pearson, Spearman, or Kendallâs Tau, based on the nature of your data.
- Apply the Formula: Each method has its unique formula. For Pearson's, the formula is:
- Compute the Coefficient: Carry out the necessary arithmetic, paying close attention to the order of operations to avoid errors.
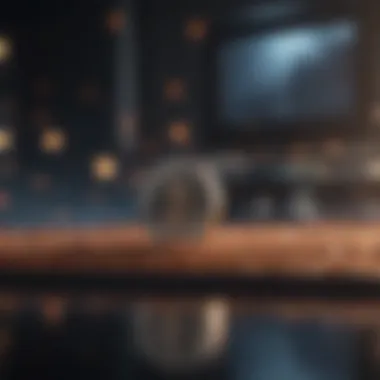
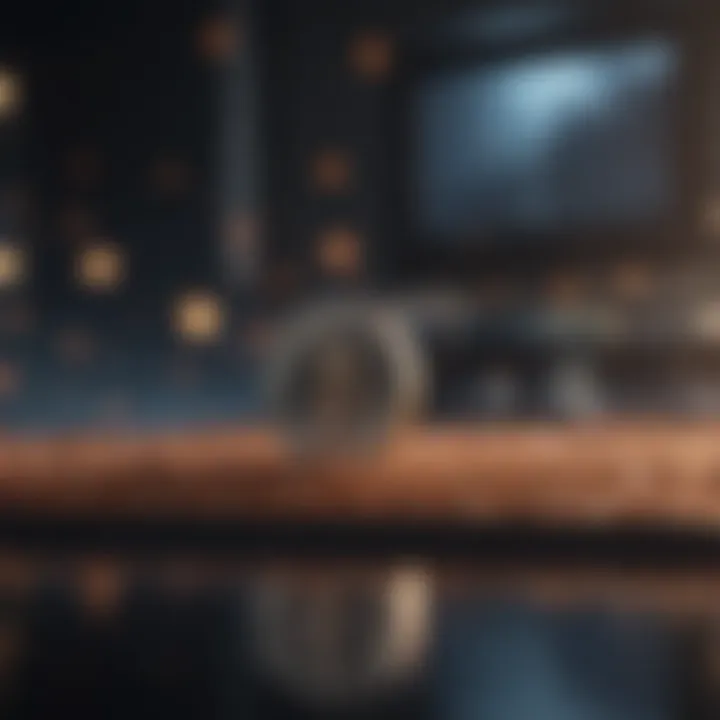
Interpreting the Results
Interpreting the results is where analytical skills truly shine. The outcome of your calculation will yield a correlation coefficient ranging from -1 to 1. Here's what that means
- +1 indicates a perfect positive correlation, suggesting that as one variable increases, the other does too.
- 0 suggests no correlation; the variables are independent.
- -1 signals a perfect negative correlation, where one variable's increase corresponds with a decrease in the other.
When presenting your findings, use visual aids like scatter plots to illustrate these relationships clearly. This not only encapsulates the quantitative findings but adds a layer of comprehension to those who may not well grasp statistical language.
A well-calculated correlation can guide decisions, but it is crucial to remember - correlation does not imply causation.
By following this step-by-step calculation process, one can ensure a structured, methodical approach to understanding the relationships between variables and drawing actionable insights.
Applications of Correlation in Finance
Correlation analysis serves as a backbone in financial decision-making. It provides insights into how two variables move in relation to each other, aiding investors in making informed choices. Understanding these applications can significantly enhance oneâs investment strategy and risk management processes. In finance, some key applications of correlation involve effective portfolio management, risk assessment, and market analysis.
Portfolio Management
In the realm of portfolio management, utilizing correlation helps investors diversify their holdings effectively. By selecting assets with low or negative correlations, an investor can reduce overall portfolio risk. For instance, if two stocks tend to move in opposite directions, one might perform well when the other falters, which cushions against market volatility.
Investors often look into the historical correlation of asset pairs to make prudent choices. Consider a scenario where someone has both technology and utility stocks. While tech stocks usually exhibit high volatility, utility stocks typically remain stable. In periods of market uncertainty, technology stocks might suffer, whereas utility stocks could hold steady. Therefore, balancing these assets based on their correlation can create a more resilient portfolio.
Risk Assessment
Risk assessment is another area where correlation plays a vital role. Understanding how different assets respond to the same market conditions can illuminate potential vulnerabilities within a portfolio. When evaluating risk, financial analysts often employ correlation matrices to visualize relationships between various assets.
For example, during economic downturns, certain sectors like consumer goods and healthcare may be negatively correlated with luxury goods sectors. If an investor allocates too much in luxury stocks without understanding this correlation, they could face a severe hit in downturns. Thus, by assessing correlations, investors can position themselves strategically against potential risks.
Market Analysis
When it comes to market analysis, correlation can uncover insights that are not readily apparent. Tracking correlations between different economic indicators can reveal trends that guide investment strategies. For example, if an investor notices a strong positive correlation between consumer spending and retail stock prices, they might consider increasing their investments in retail stocks when consumer spending rises.
Additionally, correlation can aid in identifying leading and lagging indicators in economic cycles. For instance, if a rise in housing starts correlates with future increases in consumer spending, both traders and analysts might adjust their strategies based on that relationship.
"Using correlation effectively can be the difference between a good investment and a great one."
In summary, correlation analysis is an indispensable tool in finance, providing insights that help optimize decisions in portfolio management, enhance risk assessment, and facilitate informed market analysis. By grasping the nuances of this statistical measure, investors can navigate the complexities of financial markets with a sharper understanding.
Common Misconceptions About Correlation
In the realm of statistics, particularly in the analysis of relationships between variables, there exist a number of misconceptions about correlation that can lead to misinterpretations and misguided decisions. Understanding these misunderstandings is essential for anyone looking to delve deeper into data analytics, especially in financial contexts.
Commonly held beliefs surrounding correlation can often overshadow more nuanced analysis that a financial analyst or an investor might consider. Addressing these misconceptions means not only sharpening analytical skills but also honing the ability to critically evaluate data before making any investment decisions.
Correlation vs. Causation
One of the most frequently encountered misunderstandings in correlation analysis is the belief that correlation implies causation. Just because two variables show a strong correlation doesnât mean that one causes the other. For instance, consider the relationship between ice cream sales and drowning incidents. When the temperatures rise, you might notice both ice cream sales and drowning incidents increase. However, it would be illogical to conclude that selling ice cream leads to more drownings; rather, both are influenced by a third variable: heat.
The key takeaway is that correlation can suggest possible relationships, but it does not establish a cause-and-effect scenario. Relying solely on correlation can be risky in decision-making. Investors need to tread carefully and consider other factors that might be at play.
Overlooking Non-Linear Relationships
Another common pitfall is a narrow focus on linear correlation while overlooking the potential impact of non-linear relationships. Linear correlation assumes a straight-line relationship, but thatâs not always the case in real-world data. For example, consider the relationship between the amount of physical exercise and body fat percentage. Initially, increased exercise will greatly reduce body fat, but after a certain point, the rate of fat loss might slow down. This creates a curve in the data that a linear correlation would fail to capture.
Knowing the limitations of correlation coefficients is crucial. Analysts should explore various methods like polynomial regression or scatter plots to identify these non-linear relationships. This broader perspective can substantially improve investment strategies and outcomes.
"Always question statistics. They can tell a story, but only if you understand the plot twists."
Limitations of Correlation Analysis
When it comes to analyzing data, understanding the limitations of correlation analysis is just as crucial as grasping its advantages. While correlation can provide insights into relationships between variables, its application comes with numerous nuances and potential pitfalls that anyone involved in data interpretation should recognize. A thorough comprehension of these limitations can aid investors, financial analysts, and students in making informed decisions based on accurate interpretations and cautious assumptions.
Data Quality Issues
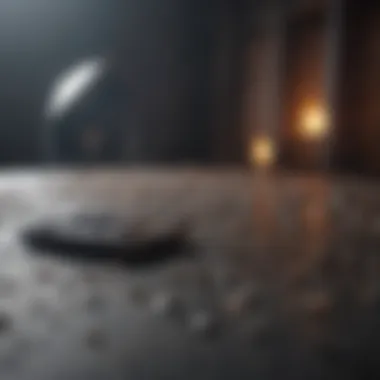
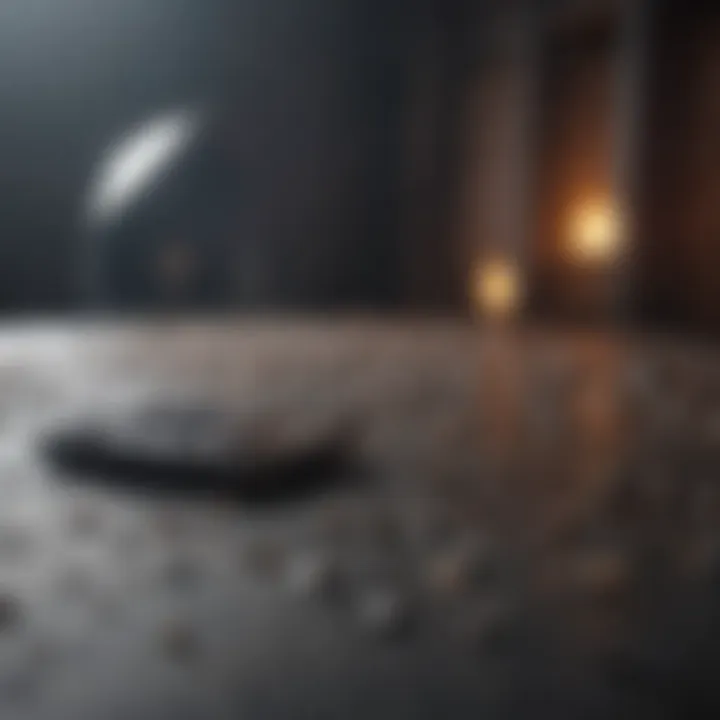
The efficacy of correlation analysis heavily relies on the quality of the data used. Data quality issues can lead to misleading results. If the data comprises outliers or errors, these anomalies can skew the correlation coefficient significantly, producing a false sense of relationship between the variables. For example, if one is analyzing the correlation between advertising expenditure and sales, but the data reflects exaggerated sales figures due to misreported inputs, the correlation may show a stronger link than actually exists.
To mitigate this risk, it's imperative to ensure that the data is clean and representative. Common data quality issues can include:
- Inaccurate recordings: Human error in data entry can distort values.
- Incomplete data: Missing values can not only reduce the sample size but also impact the overall analysis, leading to biased results.
- Non-representative samples: Using a data set that doesnât accurately represent the population can give an erroneous picture of correlation.
In essence, before delving into any correlation analysis, verifying and validating the data is a fundamental task. Regular audits and implementing stringent data collection protocols help assure that the outcomes reflect reliable inter-variable relationships rather than garbage-in-garbage-out scenarios.
Sample Size Considerations
When discussing correlation, sample size is another critical factor that cannot be overlooked. A small sample size can generate results that are not only unreliable but also potentially misleading. This is especially true in the realm of finance and investments, where decisions are often made based on marginal differences in correlation coefficients.
Smaller data sets tend to have higher variance and can lead to larger confidence intervals, making it increasingly difficult to discern whether an observed correlation is genuine or simply a product of random chance. A glance at typical sample size considerations might reveal:
- Statistical significance: A larger sample size enhances the reliability of the correlation findings, making it easier to achieve statistical significance.
- Type I and Type II errors: With limited data, there's a higher risk of both false positives and false negatives, complicating the understanding of variable relationships.
- Generalizability: Findings derived from small samples often lack broader applicability, inhibiting analysts from confidently extending these results to the wider population.
Thus, a thoughtful approach to sample sizeâfrom preliminary analysis to final interpretationâensures that correlation assessments maintain their integrity and are applicable in real-world contexts.
As such, being aware of these limitations empowers analysts to tread carefully when analyzing correlations, ensuring they don't draw conclusions based on flawed or misrepresentative data.
"Correlation does not imply causation, but caution in correlation analysis implies wisdom."
Ultimately, the essence of correlation analysis lies not only in the conclusions drawn but also in recognizing its constraints, allowing for decisions grounded in both insight and realism.
Practical Examples and Case Studies
Exploring real-world scenarios where correlation plays a significant role adds a layer of understanding to the theoretical aspects. These practical examples and case studies are not just academic exercises; they serve as critical tools for financial enthusiasts and individual investors alike. By delving into how correlation is applied across different sectors, readers can grasp its tangible benefits and the considerations that need to be taken into account when interpreting results.
Using correlation in practical settings can offer insights that purely theoretical models may not capture. For instance, tracking the relationship between two distinct stocks can reveal underlying market dynamics that could lead to strategic investment decisions. Moreover, understanding how various economic indicators correlate assists investors in predicting market movements and overall economic health. This link between theory and practice proves essential for anyone looking to enhance their analytical prowess in financial contexts.
Correlation in Stock Analysis
In the realm of stock analysis, correlation can be a double-edged sword. On one hand, it provides investors with a powerful lens through which to evaluate potential investments. For instance, consider an investor looking at the correlation between the performance of tech giants, like Apple and Microsoft. If these companies show a high positive correlation (close to +1), it could imply that they often move in tandem due to similar market conditions or consumer behavior. This knowledge can help an investor make informed decisions on portfolio diversification.
Conversely, failing to account for correlation can lead to unexpected pitfalls. Imagine an investor who heavily invests in two stocks that are highly correlated; they might inadvertently increase their exposure to a specific sector downturn. Therefore, understanding correlations allows for calculated risks and better risk management in portfolio construction.
Key Considerations in Stock Correlation
- Sector Performance: Stocks within the same industry may show high correlation due to shared drivers.
- Market Trends: Economic cycles can create temporary relationships between seemingly unrelated stocks.
- Time Frames: Daily, weekly, or monthly correlations can differ markedly due to short-term volatility.
"Correlation does not imply causation, yet it often can paint a significant part of the picture for investors."
Correlation in Economic Indicators
Economic indicators, such as unemployment rates, inflation, and GDP growth, offer a wealth of information when analyzed through the lens of correlation. Understanding how these indicators interact provides investors insight into the health of an economy and the potential performance of the markets. For example, a rise in consumer confidence often correlates with increased retail sales; recognizing this may lead to bullish sentiment in retail stocks.
Analyzing the correlation between interest rates and stock market performance can also uncover trends that might not be immediately apparent. As interest rates rise, borrowing costs increase, which can lead to reduced consumer spending and, consequently, lower earnings for companies. By identifying these relationships, investors can better anticipate market shifts and adjust their strategies accordingly.
Practical Implications of Economic Correlation
- Predictive Analysis: Understanding the interplay between indicators helps in forecasting economic trends.
- Investment Strategies: Investors can develop tailored strategies based on how indicators correlate and subsequently impact the markets.
- Policy Understanding: Correlation analysis fosters a deeper grasp of fiscal and monetary policies and their ramifications on investments.
The Future of Correlation Analysis
As we continue to navigate an era defined by rapid advancements in technology, the future of correlation analysis is set to evolve significantly. This section emphasizes the escalating importance of correlation analysis in various fields, particularly in finance, where data-driven decision making is becoming paramount. The growing availability of big data alongside sophisticated analytical tools is transforming how investors and analysts approach correlation, enhancing their capacities to understand and predict market behaviors.
Advancements in Technology
The digital landscape is changing at a breakneck pace. With tools and software like MATLAB, R, and Python finding a home in everyday analysis work, the task of calculating correlation has never been easier or more precise. Machine learning models are also playing a pivotal role in refining methods of analyzing correlation relationships within vast data sets.
- Automation: Automation of data collection and analysis means researchers can work more efficiently, focusing on drawing insights rather than sifting through raw data. For instance, automated scripts can pull data from multiple financial markets, calculate correlation coefficients, and update reports in real-time.
- Artificial Intelligence: AI is paving the way for nuanced analysis. AI systems can identify patterns and correlations that may not be visible to the naked eye. They can also adapt over time, improving accuracy based on new data.
- Cloud Computing: The advent of cloud platforms has democratized access to sophisticated analytical tools. Investors can now utilize these shared resources without the burden of extensive infrastructure, making high-level analysis more accessible.
Evolving Methodologies
Methodological advancements in correlation analysis are another key aspect driving its future. Traditional metrics alone may no longer suffice in an age where datasets are complex and multifaceted.
- Multivariate Analysis: Instead of examining just two variables, the trend is moving toward multivariate analyses which can provide a more holistic view. This means looking at how multiple variables interact with one another, yielding richer insights. For example, instead of just correlating stock prices with interest rates, analysts might now consider inflation rates, geopolitical events, and consumer spending habits.
- Bayesian Statistics: This approach is becoming more popular, as it allows for the incorporation of prior knowledge into the analysis, which can refine correlations over time and yield better forecasts.
- Network Analysis: An emerging methodology that examines the relationships and interdependencies among various entities. In finance, for instance, instead of just plotting stock correlations, analysts could create networks to see how one stock might influence another through direct or indirect connections.
"Understanding these new methodologies is crucial for any analyst who wishes to stay ahead in an increasingly competitive field."