Exploring Decision Support Systems in Investment Strategies
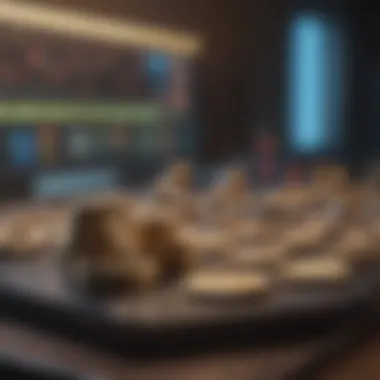
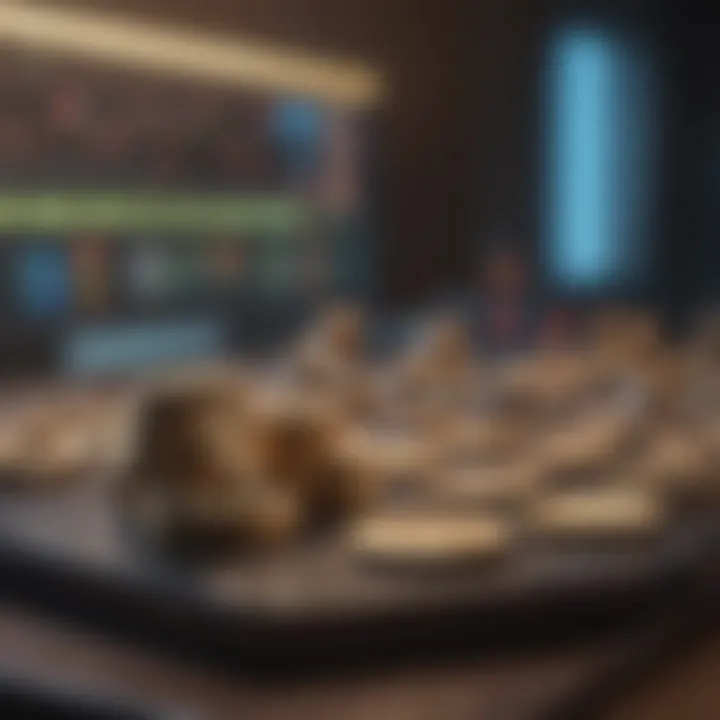
Intro
In today’s rapidly transforming financial landscape, the ability to make informed investment decisions can set successful investors apart from others. Decision Support Systems (DSS) emerge as a powerful ally, offering analytical tools that can refine and bolster investment strategies. As individuals seek ways to navigate the complex world of finance, understanding the nuances of DSS becomes essential.
Decision Support Systems are not merely tools; they serve as frameworks—tailored to process, analyze and project various types of data that are crucial to investment decisions. From budding investors to seasoned professionals, leveraging DSS can make the difference between a well-calibrated portfolio and one that flounders. The integration of technology into investment processes is more than a trend; it’s a shift in how we approach finance itself.
Moving forward, the exploration of DSS will reveal numerous facets, including their types, key functionalities and their impact on investment strategies.
Overview of the Financial Product
Decision Support Systems encompass a broad spectrum of applications focused on assisting in the decision-making process but especially shine amidst investment strategies.
Description of the product
At its core, a Decision Support System integrates data from multiple sources, providing investors with tools to evaluate options and predict outcomes. These products can range from advanced data analytics platforms to simpler dashboard tools that aggregate information and generate reports. Each system is designed to analyze diverse data sets, whether financial trends, market movements, or socio-economic factors, and translate them into actionable insights.
Key features and benefits
- Data Integration: DSS pulls together data from several sources, ensuring investors have a holistic view of the market.
- Predictive Analytics: Advanced models utilize historical data to forecast future trends or assess potential risks.
- User-friendly Interface: Most DSS products come with intuitive interfaces that allow even non-technical users to navigate complex data without feeling overwhelmed.
- Customization: These systems can be tailored to meet the specific needs of individual investors or firms, ensuring that the right metrics are highlighted.
The benefits extend far beyond mere ease of use; DSS supports enlightened decision-making by allowing investors to:
- Minimize risk through data-driven insights.
- Make timely decisions that capitalize on market opportunities.
- Monitor performance in real-time.
"In the dynamic world of finance, staying ahead often requires insights that only advanced analytical systems can provide."
Investment Strategies and Tips
Understanding how to effectively use tools like DSS goes hand-in-hand with the formulation of robust investment strategies.
Recommended investment approaches
- Diversification: Leverage the analytical capabilities of DSS to identify potential investment opportunities in varied sectors.
- Value Investing: Use predictive analytics to pinpoint stocks that may be undervalued or poised for growth.
- Momentum Trading: Apply real-time data feeds to catch rising trends early, enabling timely entry points into trades.
Risk assessment and management strategies
Investments invariably involve risk. Employing DSS can help manage these uncertainties effectively by:
- Utilizing scenario analysis to contemplate various market situations and their impact on investments.
- Establishing risk parameters based on historical data, providing a clearer picture of potential downsides.
- Continuously monitoring market changes, adjusting strategies in response to evolving conditions.
By understanding and applying the insights facilitated by Decision Support Systems, investors can navigate the complexities of modern financial markets with greater confidence and effectiveness.
Ultimately, these systems represent a convergence of technology and finance—a synthesis that empowers investors to make choices that not only maximize returns but also align with their financial goals.
Prolusion to Decision Support Systems
In the intricate realm of finance, where decisions can make or break fortunes, the art of decision-making plays a pivotal role. That's where Decision Support Systems, or DSS, come into play. Without a doubt, these systems are more than just technological innovations; they embody a strategic blend of data analysis and user-friendly interfaces that enable investors to make informed choices. The importance of understanding DSS cannot be overstated, especially for individual investors and financial enthusiasts aiming to navigate a complex investment landscape.
At its core, a Decision Support System assists investors in analyzing large sets of data. It allows for the synthesis of various data points into coherent information that informs investment strategy. Imagine having an intelligent assistant that digests hundreds of reports, market trends, and forecasts, right at your fingertips. This capability essentially transforms data into actionable insights, enhancing the decision-making process.
Benefits of Understanding DSS
- Data-Driven Insights: DSS leverages data analytics to provide insights that may not be visible to the naked eye, helping to uncover patterns and trends.
- Improved Risk Management: With DSS, potential risks can be quantified and assessed earlier in the decision-making process.
- Time Efficiency: Automating analysis saves time, allowing investors to focus on strategy refinement rather than nugging through piles of data.
- Customization: Many systems offer customizable features, enabling users to tailor dashboards and reports to suit their specific investment goals.
Considerations About DSS
However, it's important to remember that utilizing DSS is not without its challenges. Data quality remains a concern; after all, garbage in, garbage out. Adequate training is also needed for users to fully leverage these tools, as they vary widely in complexity. Furthermore, financial investors must be cautious about their reliance on these systems, ensuring that human intuition and expertise remain a part of the decision-making puzzle.
In essence, understanding Decision Support Systems offers a clear path toward enhancing investment strategies. Grasping this concept is like holding a set of keys that unlock doors to informed decision-making and smart investments. It's imperative for investors, both rookies and veterans alike, to adopt these systems and reap the rewards they present.
Core Components of Decision Support Systems
The core components of Decision Support Systems (DSS) play a vital role in shaping effective, informed investment strategies. As investors grapple with an ever-increasing amount of data, understanding how these components interact can enhance the decision-making process significantly. Dost upwards seems like the best plan of action - without a solid understanding of the core components, investors might find themselves lost in a fog of information. Let's dissect the main components: Data Management, Model Management, and User Interface, each of which adds its unique flavor to the DSS stew.
Data Management
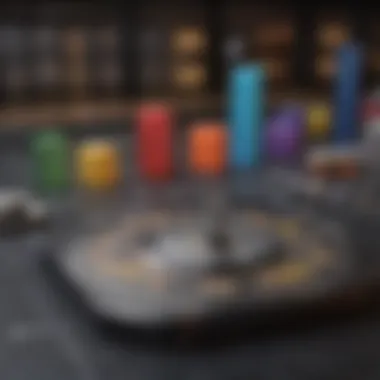
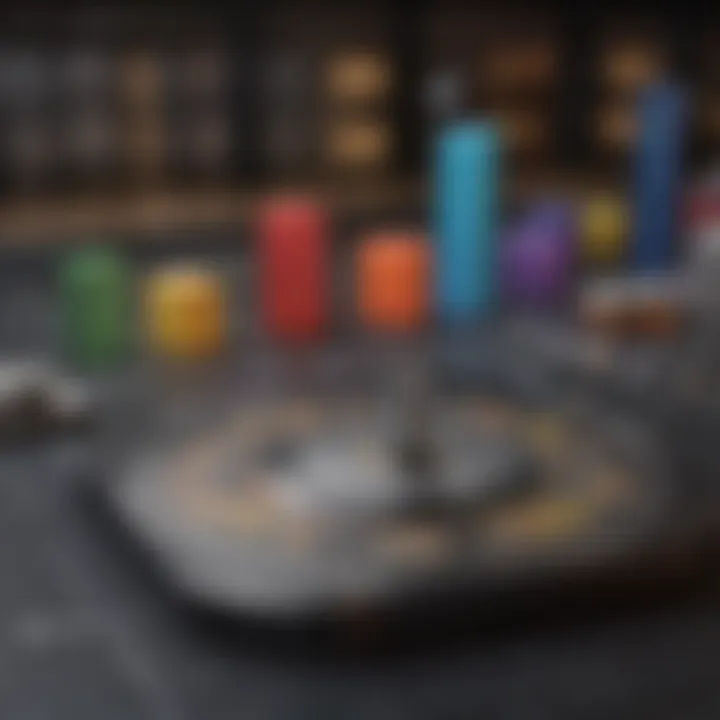
Data management is at the heart of any Decision Support System. It involves the collection, storage, and analysis of data and ensures that high-quality information is readily available for older systems as well as the newer, more advanced ones. Investors often encounter vast datasets from various sources: market trends, economic indicators, historical data, and more. Managing this data effectively is crucial.
- Collection: Efficient data collection methods are necessary for gathering relevant information. Techniques can vary from automated data scraping on financial news websites to using APIs for real-time market data. The goal here is to have a rich pool of data that can inform decisions.
- Storage: Once data is collected, it must be stored securely. Databases must allow for quick retrieval and analysis, whether that be through SQL databases or more modern NoSQL options.
- Analysis: Ultimately, it's not just about having data, but interpreting it. Analytical tools and models process this information to extract insights that guide investment strategies. For instance, a well-designed data management component can lead to timely alerts about stock price changes, enabling investors to act before prices fluctuate severely.
Robust data management promotes accuracy and reliability, which in turn fosters confidence in the system’s recommendations.
Model Management
Model management refers to the frameworks used to simulate various investment scenarios and predict outcomes. Having effective models in place is like having a compass in the investment jungle, guiding strategies toward success. Here’s why model management holds importance:
- Model Development: Creating investment models requires deep financial knowledge and an understanding of market behavior. Models could range from statistical methods to machine learning algorithms that predict market movements based on historical patterns.
- Model Selection: Not all models suit every situation. Choosing the right model to apply for specific investment strategies calls for careful consideration. For example, contextual models may analyze macroeconomic factors while transactional models can focus on specific stock movements within set intervals.
- Model Maintenance: The financial landscape changes, and so too must the models we employ. Regular updates and back-testing against real-world conditions are critical. Adjustments ensure the models reflect current market dynamics, thus improving their predictive power.
The strength of model management lies in its ability to simulate outcomes and assess the risks and rewards associated with different investment strategies.
User Interface
The user interface (UI) serves as the bridge between investors and their decision support systems. A well-designed UI can lead to swift interpretation of complex data, assisting investors in making decisions quickly, akin to having a trusty GPS guiding them through unknown territory.
- Ease of Use: A user-friendly interface is imperative. Ideally, it should offer intuitive navigation and present information in a digestible format, favoring visual tools like charts and graphs over dense text. Someone's who not a tech genius should still feel comfortable using it.
- Customization: Different investors have varying needs and preferences. A flexible UI allows users to tailor views, reports, and dashboards according to personal or professional requirements, ensuring relevant data is always at their fingertips.
- Integration: The UI should seamlessly integrate with other systems and datasets, providing a holistic view. For investors operating on multiple platforms or using varying data sources, this functionality becomes indispensable.
In essence, the user interface is crucial in enabling smooth interactions with the DSS, simplifying complex concepts, and empowering users to make informed decisions quickly.
The Core Components of Decision Support Systems—Data Management, Model Management, and User Interface—are essential for optimizing investment strategies, accommodating a wealth of information, simulating outcomes, and facilitating ease of use.
Understanding these components helps investors leverage DSS effectively, transforming raw data into actionable insights.
Types of Decision Support Systems
In the realm of investment strategies, understanding the different types of Decision Support Systems (DSS) is crucial for investors aiming to refine their decision-making processes. Each type of DSS offers unique advantages that can significantly affect how individuals and organizations analyze data, assess risks, and enhance overall investment outcomes.
Data-Driven DSS
Data-driven DSS are predominantly focused on areas rich in data collection and processing. They provide the tools to sift through massive datasets and extract relevant insights that inform investment decisions. For instance, imagine an investor tasked with evaluating various stocks based on historical performance and social sentiment. A data-driven DSS could pull data from sources as varied as stock market trends, social media sentiment, and economic indicators to create a consolidated view. This approach allows users to make informed decisions backed by quantitative facts and analytics. Such robust systems enable investors to:
- Identify trends and patterns in large datasets to spot potential investment opportunities.
- Automate the data collection process to save time and minimize human error.
- Visualize data through dynamic dashboards for easier interpretation.
Data-driven DSS is especially beneficial in high-velocity markets where real-time data influences investment strategies.
Model-Driven DSS
Model-driven DSS play a pivotal role in offering a structured environment for applying mathematical and statistical models to investment decisions. These systems utilize complex algorithms that can simulate various scenarios based on a multitude of variables. For example, a model-driven DSS may allow investors to assess potential outcomes of different investment choices under varying market conditions. The systems can include:
- Predictive models that forecast financial outcomes based on historical datasets.
- Optimization models that help in selecting the best portfolio allocations to maximize returns while controlling for risks.
- Simulation frameworks to evaluate different market scenarios and their potential impacts on investments.
Utilizing model-driven DSS can give investors a competitive edge by enabling them to approach investment strategies with a scientifically-backed mindset.
Knowledge-Driven DSS
Knowledge-driven DSS revolve around the use of expert systems that encapsulate knowledge from various fields to enhance decision making. These systems are designed to simulate human reasoning in investment scenarios, drawing from best practices, market expertise, and analytical insights accumulated over time. A prime example could be a system that analyzes past asset performance in relation to macroeconomic indicators to provide investment recommendations. Generally, knowledge-driven DSS might help investors:
- Leverage historical lessons and expert insights for current investment strategies.
- Integrate qualitative data such as expert opinions with quantitative data, providing a holistic view of potential investments.
- Personalize recommendations by considering the investor's risk tolerance and financial goals.
Overall, knowledge-driven DSS can be incredibly effective for those looking to marry human intuition with data-backed decision-making.
As investors navigate the complexities of the financial landscape, being well-versed in these types of Decision Support Systems enables them to approach their strategies with greater confidence and clarity. The intelligent interplay of data, models, and expert knowledge can help in designing resilient investment portfolios that stand the test of market fluctuations.
Role of DSS in Investment Strategies
Decision Support Systems (DSS) are vital tools for investors aiming to enhance their decision-making processes. These systems facilitate more informed investment choices by providing a structured framework for data analysis, risk management, and strategic planning. In a world where financial markets are in constant flux, the ability to leverage sophisticated analytical capabilities becomes a game-changer.
Enhancing Analytical Capabilities
One of the standout features of DSS is their ability to transform raw data into actionable insights. Investors are faced with an abundance of information—from market trends to financial statements. Harnessing this data efficiently requires a level of analysis that is both deep and nuanced. A well-designed DSS can integrate various data sources, allowing investors to evaluate their options thoroughly.
This goes beyond mere number crunching. Advanced analytics can uncover patterns that might not be immediately obvious. For example, a hedge fund might use a DSS to analyze macroeconomic indicators alongside specific stock performances. The result? A clearer picture of potential investment opportunities that align with their risk tolerance and strategic goals.
In practice, this might look like an investor employing a model-driven DSS that simulates different investment scenarios based on historical data. They might, for instance, assess how a shift in interest rates could affect their current portfolio allocations. This capability for analysis makes DSS an indispensable resource for serious investors.
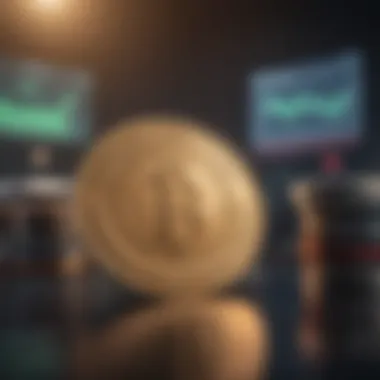
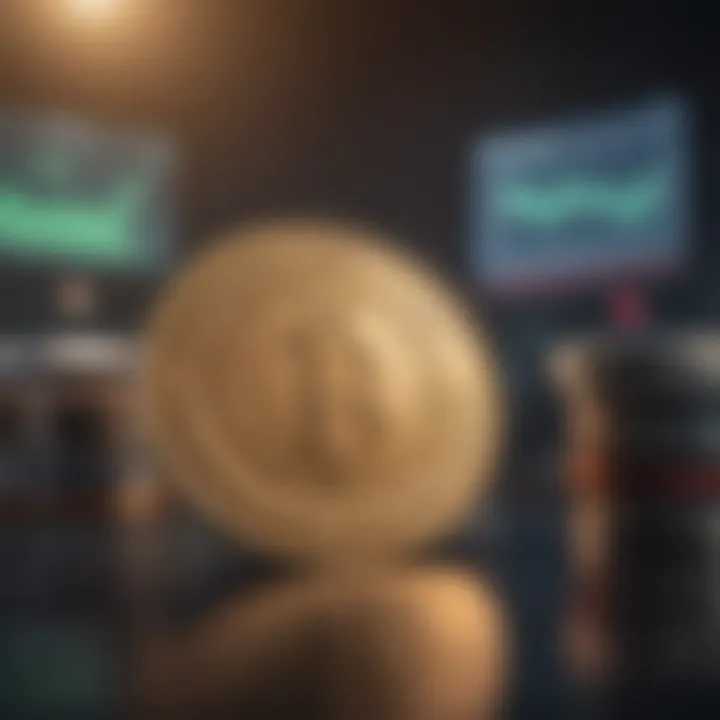
Risk Assessment and Management
Investment inherently comes with risk, and understanding it is crucial for effective financial planning. DSS can help investors identify, quantify, and mitigate risks associated with their investment strategies. With the right tools, one can visualize potential pitfalls and gauge the probable impact of various risk factors—be it economic downturns, regulatory changes, or market volatility.
Consider a real-life example where a diversified investment firm employs a knowledge-driven DSS. This system pulls from vast databases and applies algorithms to assess the risk profile of asset classes. It can alert managers to potential red flags—like a sudden spike in volatility for a particular sector—allowing for proactive adjustments in their strategies.
Moreover, a robust DSS offers scenario analysis to forecast potential losses under varying circumstances. This capability ensures that investors aren’t caught off guard and can make educated decisions based on an analysis of risk factors. By adopting a data-driven approach, they can optimize their portfolios while balancing expected returns against risk.
Scenario Analysis
Scenario analysis is a critical component of what DSS provides, enabling investors to simulate future market conditions and their possible effects on investments. This aspect of decision-making is essential, especially in volatile markets where the stakes can be extremely high.
An example of this would be a retail investor contemplating an investment in a technology stock. By utilizing a DSS, they can create scenarios that reflect different future states of the market—like a drastic technological advancement or regulatory changes impacting data privacy. Each scenario can present different end results, helping investors to gauge the likelihood and implications of each.
This proactive approach can help in strategizing alternative investment paths. For example, if the analysis reveals that a regulatory change may negatively impact a primary investment, the investor might diversify their portfolio or shift focus to less vulnerable sectors. The ability to analyze various futures equips investors not just to react, but to anticipate.
"A well-implemented Decision Support System doesn't just clarify paths; it reveals new roads entirely."
Consequently, DSS plays a transformative role in enhancing analytical capability, managing risk effectively, and facilitating in-depth scenario analysis in investment strategies.
In summary, Decision Support Systems offer invaluable support to investors navigating the complex landscape of financial markets. By transforming data into actionable insights, assessing risk effectively, and allowing for comprehensive scenario analysis, these systems can significantly enhance the decision-making process. For those looking to optimize their investment strategies, leveraging DSS is not just beneficial; it’s essential.
Technological Advances Supporting DSS
In the rapidly shifting landscape of finance, the role of technology cannot be overstated. Decision Support Systems (DSS) harness the power of contemporary technological advancements to enhance their functionalities. The integration of innovative tools not only streamlines decision-making processes but also elevates the accuracy and effectiveness of investment strategies. As investors grapple with increasing volumes of data, understanding the technological advancements supporting DSS becomes crucial.
Artificial Intelligence and Machine Learning
Artificial Intelligence (AI) and Machine Learning (ML) have revolutionized the way data is analyzed and interpreted within DSS. With the capacity to analyze patterns and identify trends, these technologies enable investors to make informed decisions based on sophisticated algorithms. For instance, ML can predict future market behavior by learning from historical data, thereby providing insights that human analysts might overlook.
- Enhanced Decision-Making: AI empowers DSS to suggest optimal investment options by evaluating complex datasets, providing tailored recommendations based on individual risk profiles.
- Automation of Routine Tasks: Routine analysis tasks that used to consume hours or even days can now be automated, freeing up valuable time for investors to focus on strategy and execution.
- Personalization: With AI, DSS can adapt to the unique preferences of investors, learning their habits to enhance user experience.
"In any financial market, the competitive edge often lies within the data. Harnessing AI in DSS allows for efficient sorting and analysis of this data, creating an environment for informed predictions and strategies."
Big Data Analytics
The deluge of data available in today’s market is astounding. Big Data Analytics empowers DSS to process vast amounts of information from diverse sources, such as social media trends, international news, and economic indicators. This capability is vital for investors seeking to stay ahead of market dynamics.
- Real-Time Analysis: DSS can deliver real-time insights, allowing investors to react swiftly to market changes, an essential feature in high-frequency trading environments.
- Data Visualization: Utilizing sophisticated visual tools, DSS can present data in an understandable and actionable format, making it easier for investors to digest critical information at a glance.
- Predictive Analytics: Through big data techniques, investors can uncover hidden patterns that may signal promising investment opportunities or emerging risks, guiding strategic decisions with a sharper focus.
Cloud Computing Integration
Cloud computing offers a flexible infrastructure that enhances the capabilities of DSS. By storing data remotely and enabling access from anywhere, it breaks down barriers that previously hindered collaboration and data sharing. Investors benefit from this model in several ways.
- Scalability: Cloud solutions allow DSS to easily scale as the demands of the investment landscape fluctuate, accommodating growth without significant expense.
- Cost Efficiency: Moving DSS to the cloud can significantly reduce costs related to hardware and maintenance, allowing firms to allocate resources toward more valuable initiatives.
- Collaboration: Cloud-based DSS facilitate real-time collaboration among investment teams, regardless of geographic location, ensuring that all members have access to the latest data and insights.
In summary, the technological advances that support Decision Support Systems, particularly AI, big data analytics, and cloud computing, play a pivotal role in reshaping investment strategies. These tools not only enhance operational efficiency but also empower investors to make informed decisions in an increasingly complex environment.
Challenges in Implementing DSS
Implementing Decision Support Systems (DSS) in investment strategies presents a complex array of challenges and intricacies. Recognizing these obstacles is essential for investors and institutions aiming to leverage technology effectively. Addressing these issues not only enhances the efficacy of DSS but also promotes better decision-making in financial contexts. Understanding these challenges helps stakeholders balance investment goals with technological advancements.
Data Quality Issues
Data quality remains a cornerstone of effective DSS. Poor data input can lead to unreliable analyses, skewing investment decisions in favor of incorrect information. For instance, consider a portfolio manager relying on outdated stock prices; they could, inadvertently, make suboptimal decisions that lose money. To avert such pitfalls, organizations must prioritize high-quality data collection and management processes.
Key factors affecting data quality include:
- Accuracy: Data must truly reflect the real-world condition it represents.
- Completeness: Incomplete datasets can throttle effective decision-making, leaving gaps that lead to risks.
- Timeliness: Financial data is ever-changing; if it's not up-to-date, it loses its relevance.
- Consistency: Disparate data sources must align to produce coherent outcomes.
Case Studies of DSS in Financial Decision Making
Decision Support Systems (DSS) are transforming how investment strategies are formulated and executed. By analyzing real-world applications of DSS, we can gain insights into their practical impact on decision-making processes in finance. This section dives into specific case studies that highlight how these systems play crucial roles in portfolio management, risk management, and trading systems. It’s in this analysis where we find tangible evidence that underscores the value of integrating technology into financial decision-making.
DSS in Portfolio Management
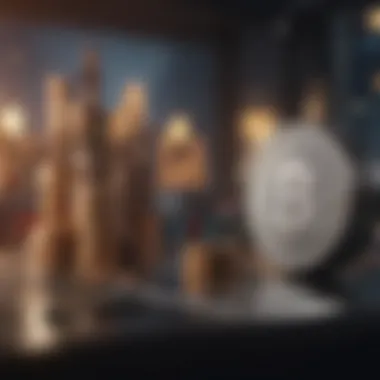
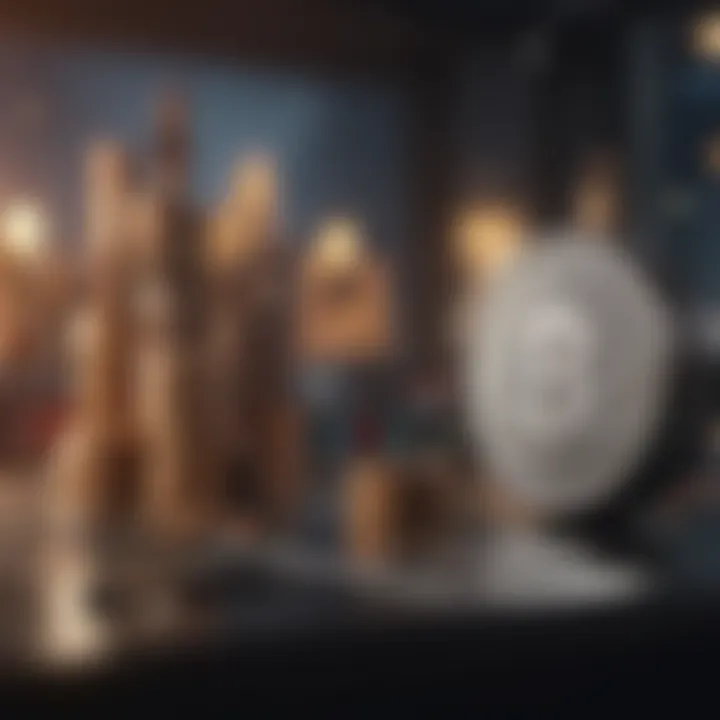
A compelling example of DSS in portfolio management is seen through major investment firms utilizing these systems to balance risk and return. For instance, imagine a firm that manages several billion dollars across various asset classes. They leverage a DSS to evaluate historical data, predictive models, and real-time market conditions, allowing portfolio managers to make informed decisions about asset allocation.
Key elements of effective portfolio management via DSS include:
- Analytical Tools: These applications enable managers to simulate various investment scenarios, assessing potential outcomes before actual investments are made. This foresight helps avoid costly mistakes.
- Diversification Strategy: DSS facilitate the balancing of portfolios by analyzing correlations between asset classes, which is essential for risk management. This approach allows for a robust investment strategy, mitigating unexpected downturns.
- Performance Tracking: Using advanced tracking measures, DSS helps investment firms continuously monitor the performance of investments against benchmarks, enabling timely adjustments.
By utilizing such systems, firms not only enhance efficiency but also increase their probability of success in fluctuating markets.
DSS in Risk Management
In the realm of risk management, DSS applications play an indispensable role in identifying, analyzing, and mitigating potential financial risks. A notable example is how energy companies employ DSS for market volatility assessments, particularly in commodities trading.
Some pivotal aspects include:
- Real-Time Risk Assessment: Utilizing live market data, DSS can analyze shifts in energy prices and predict potential price spikes, enabling firms to hedge against unfavorable market conditions.
- Scenario Simulation: Firms can run simulations based on various economic factors and geopolitical events, providing insights into how these events might affect their risk profile.
- Compliance Monitoring: Maintaining regulatory compliance is critical in finance. DSS can support firms by automating the compliance checks, ensuring adherence to rules while minimizing human error.
Such capabilities make DSS invaluable for institutions looking to safeguard investments against unforeseen circumstances.
DSS in Trading Systems
One of the more dynamic uses of DSS can be found in automated trading systems. A prime example includes firms using algorithmic trading, where DSS plays a key role in executing trades based on set parameters and market conditions.
Critical factors include:
- Speed and Efficiency: Automated systems can process large amounts of data quickly, identifying trading opportunities that a human trader might overlook. This speed can lead to better prices and more profitable trades.
- Data-Driven Decisions: By relying on historical trade data and market analytics, these DSS guide traders in making informed choices, effectively using information that is often too complex for manual analysis.
- Risk Management Features: Many trading systems integrate risk management protocols via DSS, alerting traders when predefined risk thresholds are met or exceeded, thus protecting capital.
To sum up, case studies demonstrate that DSS not only enhance decision-making efficacy but also provide a strategic edge in a highly competitive field. By adopting these systems, financial institutions can harness data, improve operational processes, and better navigate the ever-changing investment landscape.
Future of Decision Support Systems
The landscape of investing is ever-changing, and the role of Decision Support Systems (DSS) is evolving right alongside it. As we gaze into the crystal ball, it’s vital to understand what the future holds for these systems in the arena of investment strategies. The significance of this very topic is manifold; as technology continues to mature, the capabilities of DSS will expand, opening new doors for investors looking to enhance their decision-making processes.
Trends in Technology
The winds of technology are blowing fiercely through the financial sector. One major trend is the integration of natural language processing within DSS, enabling these systems to understand and interpret vast amounts of unstructured data—from financial news articles to social media sentiment. Tools like Bloomberg Terminal and Thomson Reuters Eikon already harness this capability, but the future signifies a more democratized access to such advanced analytics.
Moreover, automation is on the rise. Algorithms increasingly assist investors by executing trades automatically based on pre-defined criteria. This feature reduces the cognitive load on investors, allowing them to focus on higher-level strategy rather than minute-to-minute market moves.
Other trends include the rise of mobile DSS applications, making it simpler for investors to access crucial data anytime, anywhere. The convergence of augmented reality and deep learning in DSS could transform how data visualizations are presented, allowing for a more intuitive exploration of complex data sets.
Evolving User Expectations
In a world where information moves at lightning speed, users have become more discerning about the tools they employ. Today's investors—particularly millennials and Gen Z—expect solutions that are not just functional but also user-friendly. The interface mustn't just be aesthetically pleasing; it should offer a seamless experience that enhances the decision-making process.
The market trends suggest a shift towards personalization in DSS. Investors are beginning to demand systems that consider their unique preferences and risk tolerance levels. This evolution means that decision support systems must also evolve, adapting their analytics and recommendations based on a user’s specific context. This tailoring can range from recommending investment portfolios aligned with individual ethical values to suggesting high-impact investment options based on real-time data analysis.
Regulatory Considerations
As the financial landscape morphs, regulatory frameworks around DSS will undoubtedly adapt. With the increasing reliance on technology comes the responsibility to ensure these systems operate within ethical boundaries. This is particularly true for algorithms that offer investment advice, as they can inadvertently propagate bias if not carefully monitored and adjusted.
Regulatory bodies are likely to ramp up scrutiny on how these systems gather data, make decisions, and behave under various market conditions. Investors should remain vigilant in understanding the compliance policies that will likely shape the evolution of DSS. Knowledge of these regulations not only safeguards investors but also drives home the value of transparency and trust in an increasingly automated world.
"The future of Decision Support Systems will be defined not just by technology, but by the ethical frameworks guiding their application and the expectations of the users who rely on them."
To wrap it up, the future of Decision Support Systems in the investment landscape will unfold with numerous exciting possibilities. By integrating evolving technologies, catering to user expectations, and keeping a keen eye on regulatory frameworks, DSS can continue to enhance the decision-making process for investors at all levels. Ultimately, as these systems will increasingly support nuanced decision-making, they will play a pivotal role in shaping the future of investment strategies.
The End
Deciding on the right investment strategy has become even more intricate in today’s fast-paced financial environment. The role of Decision Support Systems (DSS) emerges as a key player in this landscape, enhancing the way investors approach their financial decisions. In this article, we’ve taken a comprehensive look at how DSS frameworks operate, their various types, and how they apply to real-world investment scenarios.
Summarizing Key Points
To distill the essence of our discussion:
- Understanding DSS: We explored what DSS is and its historical evolution, providing a basis for grasping its practical importance.
- Core Components: The elements of data management, model management, and user interfaces are pillars of a robust DSS framework, aiding in efficient decision-making.
- Types of DSS: Recognizing the distinctions between data-driven, model-driven, and knowledge-driven systems helps tailor the decision-making process to fit specific needs.
- Investment Strategies: The systems enhance analytical capabilities, improve risk assessment, and allow thorough scenario analyses which are crucial for informed investing.
- Technological Advances: We examined how technologies like artificial intelligence and cloud computing are injecting fresh capabilities into DSS.
- Challenges: Data quality and user buy-in pose significant challenges which have to be navigate thoughtfully.
- Case Studies: Real-world examples in portfolio, risk management, and trading systems illustrate the tangible benefits of DSS in action.
- Future Trends: We also touched on the dynamics shaping the future, from emerging technologies to heightened investor expectations.
Implications for Investors
The relevance of understanding DSS cannot be overstated. For investors, the integration of these systems can mean a path towards smarter decision-making that is both analytical and intuitive. Key implications include:
- Enhanced Decision-Making: With a more analytical approach, investors can mitigate risks and seize opportunities that may have been unnoticed without such systems.
- Personalized Strategies: DSS allows customization, enabling investors to align decision-making processes with individual goals and risk tolerances.
- Stay Ahead of Trends: As financial landscapes evolve rapidly, those utilizing DSS will likely have a leg up in adapting their strategies to meet market demands.
"In the world of investing, having the right information at your fingertips isn't just an advantage; it's a necessity."